synthetic fire-smoke Computer Vision Project
Updated 2 years ago
9.9k
views481
downloadsMetrics
MSFFD
1. MSFFD Download Link
We provide two ways to access the MSFFD:
-
Roboflow, the address of this dataset is:https://universe.roboflow.com/yunnan-university/synthetic-fire-smoke
-
Cloud Drive, we provide the data in different annotation formats for different target detection algorithms (coco, darknet, yolov6, yolov7, yolov8).
-
The download links for Google Cloud Drive are: coco, darknet, yolov6, yolov7, yolov8, different_weather_and_time:.
-
The download links for baidu cloud disk are:
-
coco: https://pan.baidu.com/s/18w33GJtQar0gfOM3ocuR1A?pwd=yqv8 提取码:yqv8
-
darknet: https://pan.baidu.com/s/1jqzTn93ixUf_TXhrCRaW4w?pwd=c8dx 提取码:c8dx
-
yolov6: https://pan.baidu.com/s/1X_2mmSyAMCshnYJIOeQnYg?pwd=fk3r 提取码:fk3r
-
yolov7: https://pan.baidu.com/s/1HgC5YpEtF3VJgLt5zRD_Uw?pwd=q89a 提取码:q89a
-
yolov8: https://pan.baidu.com/s/1VDbQBMD3SamF0a7_r9aLow?pwd=2rsq 提取码:2rsq
-
different_weather_and_time: https://pan.baidu.com/s/1O4QKkeJubQa6lZKRdnwR6w?pwd=txil 提取码:txil
-
-
2. Scenario introduction
In order to achieve a realistic simulation of forest fires, we use Unreal Engine 5 to build a diverse forest scene.
Unreal Engine 5 can meet the details of real-world forest terrain, weather, time, lighting, texture, etc. It can also simulate multi-scale forest fires in different periods, which is ideal for the construction of synthetic datasets.
We built eight scenes with different terrain through Unreal Engine 5, containing three types of weather conditions: sunny, foggy, and rainy-snowy, and three different times of day, evening, and night.
The following table shows the details of the raw data.
Scenario | Resolution | FPS | Duration | Size | Weather | The number of objects | Description | |
---|---|---|---|---|---|---|---|---|
1 | a | 1480×684 | 17.6 | 126 seconds | 270.7 Mb | Sunny | Multiple Objects | Daylight, mountains, and plains |
2 | a | 1480×684 | 17.7 | 85 seconds | 227.5 Mb | Sunny | No Objects | Evening, mountains, and plains |
3 | a | 1480×684 | 17.2 | 109 seconds | 30.2 Mb | Sunny | Multiple Objects | Night, mountains, and plains |
4 | a | 1480×684 | 13.8 | 79 seconds | 184.1 Mb | Sunny | No Objects | Evening, mountains, and plains |
5 | a | 776×452 | 18.6 | 113 seconds | 75.3 Mb | Sunny | No Objects | Daylight, mountains, and plains |
6 | a | 1480×684 | 17.9 | 82 seconds | 9.7 Mb | Sunny | Single Object | Night, mountains, and plains |
7 | b | 1480×684 | 29.9 | 115 seconds | 496.2 Mb | Sunny | Multiple Objects | Daylight, mountains, and plains |
8 | b | 1480×684 | 28 | 82 seconds | 314.4 Mb | Sunny | No Objects | Daylight, Evening, Night, mountains, and plains |
9 | b | 1480×684 | 29.9 | 101 seconds | 477.4 Mb | Sunny | Single Object | Daylight, mountains, and plains |
10 | b | 1480×684 | 26.5 | 83 seconds | 43.4 Mb | Sunny | Single Object | Night, mountains, and plains |
11 | c | 1480×684 | 18.5 | 195 seconds | 258.4 Mb | Sunny | Multiple Objects | Daylight, Night, mountains, and plains |
12 | c | 1480×684 | 21.2 | 190 seconds | 423.3 Mb | Sunny | Single Object | Daylight, Evening, Night, mountains, and plains |
13 | d | 1480×684 | 9.99 | 133 seconds | 145.9 Mb | Sunny | Single Object | Daylight, Evening, Night, mountains, lakes, and plains |
14 | d | 1480×684 | 15.7 | 122 seconds | 169.2 Mb | Sunny | Multiple Objects | Daylight, Evening, Night, mountains, lakes, and plains |
15 | d | 1480×684 | 11.1 | 125 seconds | 136.5 Mb | Sunny | No Objects | Daylight, Evening, Night, mountains, lakes, and plains |
16 | e | 1280×720 | 30 | 148 seconds | 348 Mb | Sunny | Multiple Objects | Daylight, mountains, and lakes |
17 | e | 1280×720 | 30 | 97 secnds | 130.2 Mb | Sunny | Multiple Objects | Night, mountains, and lakes |
18 | e | 1280×720 | 30 | 89 seconds | 92 Mb | Sunny | Multiple Objects | Evening, mountains, and lakes |
19 | f | 1280×720 | 24.6 | 60 seconds | 342.8 Mb | Rainy&Fog | Multiple Objects | Daylight, mountains, lakes, and plains |
20 | f | 1280×720 | 23.7 | 29 seconds | 20.2 Mb | Rainy&Fog | Multiple Objects | Evening, Night, mountains, lakes, and plains |
21 | f | 1280×720 | 10.6 | 57 seconds | 6.7 Mb | Rainy&Fog | Multiple Objects | Night, mountains, lakes, and plains |
22 | f | 1280×721 | 10.6 | 36 seconds | 28.1 Mb | Rainy&Fog | Multiple Objects | Evening, mountains, lakes, and plains |
23 | g | 1280×722 | 10.2 | 99 seconds | 206 Mb | Sunny | Multiple Objects | Daylight, plains |
24 | g | 1280×723 | 10.5 | 27 seconds | 21.8 Mb | Sunny | Multiple Objects | Night, plains |
25 | g | 1280×724 | 9.3 | 61 seconds | 92 Mb | Sunny | Multiple Objects | Evening, plains |
26 | h | 1280×724 | 24.7 | 40 seconds | 251.1 Mb | Snow | Multiple Objects | Daylight, mountains, and plains |
27 | h | 1280×724 | 26 | 33 seconds | 143.4 Mb | Snow | Multiple Objects | Night, mountains, and plains |
28 | h | 1280×724 | 25.3 | 49 seconds | 183.6 Mb | Snow | Multiple Objects | Evening, mountains, and plains |
2.1 Diverse scenarios
We built eight multi-scale forest scenarios with different terrain and vegetation.
2.2 Multiple Weather
MSFFD simulates not only sunny weather scenes, but also rainy, foggy and snowy weather scenes.
2.3 Multiple times of day
MSFFD considers multimodal forest fire images at different points of the day (day, evening and night).
2.4 Different number of fire objects
In a multimodal forest scenario with multiple terrains, multiple meteorological conditions, and multiple time points, we set up three fire target number scenarios: no objects, single object, and multiple objects, aiming to fully evaluate the performance of the simulated multimodal forest fire dataset in terms of target detection algorithm performance.
3. Details of the dataset
The following table shows the distribution of the images and annotated boxes of the dataset in different weather and at different times of the day.
Multimodal scenarios | Number of images | fire | smoke | Number of annotation boxes | Average number of instances boxes per image |
---|---|---|---|---|---|
Sunny | 3292 | 8423 | 7095 | 15518 | 4.7 |
Snowy | 312 | 772 | 547 | 1319 | 4.2 |
Rainy & Fog | 370 | 432 | 494 | 926 | 2.5 |
Daytime | 2209 | 4684 | 3807 | 8491 | 3.8 |
Evening | 560 | 2052 | 1581 | 3633 | 6.5 |
Night | 1205 | 2891 | 2748 | 5639 | 4.7 |
The following table shows the size distribution of all comment boxes.
class | small boxes | medium boxes | large boxes | total |
---|---|---|---|---|
fire | 4848 | 4114 | 665 | 9627 |
smoke | 1883 | 2037 | 4216 | 8136 |
all | 6731 | 6151 | 4881 | 17763 |
The following figure shows the distribution of all annotation boxes in the dataset, as shown below.
The distribution of the centroid coordinates of the fire and smoke annotation boxes in different modes is shown in the following figure.
The size distribution of fire and smoke annotation boxes for different modes is shown in the following figure.
4. Experimental results
4.1 Experimental results of MSFFD in single-stage object detection algorithm
Model | #Param. | Flops | Weight Size | AP50:95 | AP50 | APFire | APSmoke | Precision | Recall | F1-socre |
---|---|---|---|---|---|---|---|---|---|---|
YOLOv8m | 25.9M | 78.9G | 52Mb | 51.2 | 87.3 | 88.7 | 86 | 83.7 | 86.9 | 85.3 |
YOLOv8l | 43.7M | 165.2G | 87.7Mb | 51.6 | 86.8 | 88.9 | 84.8 | 84.6 | 80.1 | 82.3 |
YOLOv8x | 68.2M | 258.1G | 136.7Mb | 47.3 | 87.1 | 88.5 | 85.8 | 84.4 | 80.2 | 82.2 |
YOLOv7 | 36.5M | 103.2G | 74.8Mb | 48.5 | 87.5 | 88.7 | 86.2 | 85.3 | 80.5 | 82.8 |
YOLOv7-X | 70.8M | 188G | 142.1Mb | 47.3 | 87.1 | 88.5 | 85.8 | 84.4 | 80.2 | 82.2 |
YOLOv6-M | 34.9M | 85.8G | 74.9Mb | 44.5 | 83.4 | 84.2 | 82.6 | 83.8 | 80.7 | 82.2 |
YOLOv6-L | 59.5M | 150.7G | 117.6Mb | 48.8 | 86.3 | 87.2 | 85.4 | 84.2 | 79.8 | 81.9 |
YOLOv6-M6 | 79.6M | 379.5G | 72.51Mb | 43.7 | 82.9 | 82 | 85.1 | 82.6 | 80 | 81.3 |
YOLOv6-L6 | 140.4M | 673.4G | 268.4Mb | 46.3 | 83.3 | 83.9 | 82.6 | 83.6 | 79.7 | 81.6 |
YOLOv5m | 21.2M | 49.0G | 42.2Mb | 45.6 | 87.1 | 88.1 | 86 | 86.6 | 81.5 | 84.0 |
YOLOv5l | 46.5M | 109.1G | 92.8Mb | 45.9 | 86.7 | 87.9 | 85.4 | 85.2 | 81.6 | 83.4 |
YOLOv5x | 86.7M | 205.7G | 173.1Mb | 45.7 | 86.3 | 87.3 | 85.3 | 84.6 | 82.3 | 83.4 |
YOLOv4 | - | 59.6G | 256Mb | - | 75.3 | 75.7 | 74.8 | 66 | 73 | 69.3 |
YOLOv4-CSP | - | 50.3G | 210.3Mb | - | 81.5 | 83.8 | 79.3 | 78 | 80 | 79.0 |
YOLOv3 | - | 65.3G | 246.3Mb | - | 82.3 | 82.6 | 82 | 83 | 77 | 79.9 |
YOLOv3-spp | - | 65.7G | 250.5Mb | - | 82.1 | 82.3 | 81.8 | 83 | 78 | 80.4 |
4.2 Experimental results in two-stage object detection algorithm
Model | Image Size | Weight Size | AP50:95 | AP50 |
---|---|---|---|---|
Faster R-CNN R50-DC5 | 640 | 1300Mb | 38.6 | 78.9 |
Faster R-CNN R50-FPN | 640 | 333.3Mb | 42.1 | 82.7 |
Faster R-CNN R50-C4 | 416 | 257.5Mb | 24.8 | 59.8 |
Faster R-CNN R101-C4 | 416 | 422Mb | 26.9 | 60 |
Faster R-CNN R101-DC5 | 416 | 1400Mb | 25.3 | 57.8 |
Faster R-CNN R101-FPN | 416 | 365.2Mb | 35.9 | 76.1 |
RPN R50 | 416 | 103.5Mb | 25.4 | 55.8 |
Fast R-CNN R50-FPN | 416 | 318.7Mb | 28.5 | 59.8 |
4.3 Experimental Results of MSFFD in Lightweight objects detection algorithm
Model | #Param. | Flops | Weight Size | AP50:95 | AP50 | APFire | APSmoke | Precision | Recall | F1-socre |
---|---|---|---|---|---|---|---|---|---|---|
YOLOv8n | 3.2M | 8.7G | 6.3Mb | 49.5 | 86.4 | 87.1 | 85.7 | 83.2 | 81.2 | 82.2 |
YOLOv8s | 11.2M | 28.6G | 22.5Mb | 50.3 | 86.4 | 87.9 | 85 | 83.1 | 81.4 | 82.2 |
YOLOv6-N | 4.7M | 11.4G | 10.0Mb | 42 | 82.2 | 82.9 | 81.5 | 83.3 | 78.8 | 81.0 |
YOLOv6-S | 18.5M | 45.3G | 10.2Mb | 44.4 | 84.1 | 85 | 83.2 | 83.9 | 80.3 | 82.1 |
YOLOv6-N6 | 10.4M | 49.8G | 21.83Mb | 42.1 | 82.1 | 82.8 | 81.3 | 83.7 | 78.9 | 81.2 |
YOLOv6-S6 | 41.4M | 198G | 85.84Mb | 43.2 | 83.1 | 83.8 | 82.5 | 83.6 | 79.4 | 81.4 |
YOLOv5n | 1.9M | 4.5G | 3.8Mb | 40.1 | 84.9 | 86.1 | 83.7 | 84.3 | 79.1 | 81.6 |
YOLOv5s | 7.2M | 16.5G | 14.4Mb | 43.7 | 86.4 | 87.9 | 84.9 | 83.7 | 80.9 | 82.3 |
YOLOv4-tiny | - | 6.8G | 23.5Mb | - | 64.5 | 51.9 | 77.1 | 80 | 58 | 67.2 |
YOLOv3-tiny | - | 5.4G | 34.7Mb | - | 71.7 | 69.6 | 73.9 | 76 | 72 | 73.9 |
4.4 Visualization of experimental results
Use This Trained Model
Try it in your browser, or deploy via our Hosted Inference API and other deployment methods.
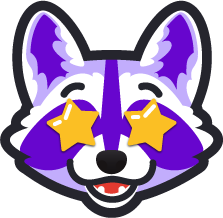
Build Computer Vision Applications Faster with Supervision
Visualize and process your model results with our reusable computer vision tools.
Cite This Project
If you use this dataset in a research paper, please cite it using the following BibTeX:
@misc{
synthetic-fire-smoke_dataset,
title = { synthetic fire-smoke Dataset },
type = { Open Source Dataset },
author = { Yunnan University },
howpublished = { \url{ https://universe.roboflow.com/yunnan-university/synthetic-fire-smoke } },
url = { https://universe.roboflow.com/yunnan-university/synthetic-fire-smoke },
journal = { Roboflow Universe },
publisher = { Roboflow },
year = { 2023 },
month = { apr },
note = { visited on 2024-12-25 },
}