Object Detection Model for Identification of Poisonous Plants in the Chesapeake Bay Watershed Computer Vision Project
Updated 2 years ago
302
views8
downloadsMetrics
Project Title Object Detection Model for Identification of Poisonous Plants in the Chesapeake Bay Watershed by Shameer Rao
Model Overview Time outside is crucial for our health, but there are risks in the great outdoors. One of the most significant issues we may encounter is poisonous plants. There isn't a singular rule to recognize them; they are not all bright red, nor do they all have three leaves. An encounter with a harmful plant could cause rashes, itching, and swelling. This object detection model will identify the four most common poisonous plants in the Chesapeake Bay Watershed, with the intended audience being American states within the Chesapeake Bay area (Delaware, Maryland, New York, Pennsylvania, Virginia, and West Virginia—and the District of Columbia). The model will have five classes; Giant Hogweed (Heracleum mantegazzianum), Poison Hemlock (Conium maculatum), Spotted Water Hemlock (Cicuta maculata), Mayapple (Podophyllum peltatum), and a null class.
Model Structure Roboflow will be used to create the model, with five classes; Giant Hogweed (Heracleum mantegazzianum), Poison Hemlock (Conium maculatum), Spotted Water Hemlock (Cicuta maculata), Mayapple (Podophyllum peltatum), and a null class to store nonessential results. I have chosen Roboflow for its in-depth analytics and various optimization tools. The smart annotation tool is one of its best features and increases workflow when annotating. Additionally, having more familiarity with Roboflow compared to Google’s Teachable Machine is also an advantage.
Data Collection Plan Each class will be trained with 100 images taken during the daytime, containing as little background noise as possible, and focused on most parts of the plants. All photos must be in JPEG or PNG format. Image size will not be an eliminating factor, but all images will be backed-up on Google Drive in case of cropping or editing the pictures. With these parameters set, I hope that the rules either eliminate or reduce bias within this model. Additionally, the images will be collected from the iNaturalist, CDC, NPS, and MD DNR websites.
Minimal Viable Product The object detection model should reach a 40.0% mAP, 50.0% precision, and 40.0% recall to be considered a success, with each class at a 50% accuracy rate as well. Although my initial benchmark is low, I aim to reach this threshold in the first or second iteration of the model. Upon reaching this threshold, the final milestone should increase up to 65.0% mAP, 75.0% precision, and 60.0% recall. These milestones should be feasible as I reached 67.2% mAP, 76.0% precision, and 61.1% recall on my second iteration of the Shark Tooth Model. I expect Giant Hogweed (Heracleum mantegazzianum), and Spotted Water Hemlock (Cicuta maculata) classes to have a lower accuracy rate due to their similarity in features. Additionally, I expect Mayapple (Podophyllum peltatum) to perform the best as it has more distinct features than the other three classes.
Use cases for this project:
Ecological Conservation: Conservationists and ecologists in the Chesapeake Bay Watershed can use the object detection model to monitor and track the spread of these poisonous plant species. By detecting their presence in various ecosystems, specialists can take appropriate measures to control their growth and prevent damage to native species.
Public Health and Safety: Local governments and parks departments can utilize this model to identify and remove poisonous plants from public spaces such as parks, hiking trails, and playgrounds. This would reduce the risk of accidental exposure to these plants, ensuring a safer outdoor environment for the community.
Agricultural Management: Farmers and landowners in the Chesapeake Bay Watershed can use the computer vision model to detect the presence of poisonous plants on their property. This would help them avoid cultivating or accidentally spreading these toxic invaders, safeguarding their crops and livestock from possible harm.
Botanical Research: Researchers studying the ecology of the Chesapeake Bay Watershed can use the object detection model to conduct large-scale surveys of poisonous plant populations in the region. This data would provide valuable information on the distribution, abundance, and interactions between these toxic species and the surrounding environment.
Environmental Education: Educators can incorporate the object detection model into educational programs to teach students and the public about poisonous plants found in the Chesapeake Bay Watershed. This would raise awareness of these hazardous species, fostering a better understanding of local ecosystems and promoting responsible outdoor behaviors.
Use This Trained Model
Try it in your browser, or deploy via our Hosted Inference API and other deployment methods.
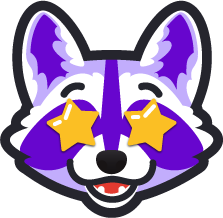
Build Computer Vision Applications Faster with Supervision
Visualize and process your model results with our reusable computer vision tools.
Cite This Project
If you use this dataset in a research paper, please cite it using the following BibTeX:
@misc{
object-detection-model-for-identification-of-poisonous-plants-in-the-chesapeake-bay-watershed-p8o4b_dataset,
title = { Object Detection Model for Identification of Poisonous Plants in the Chesapeake Bay Watershed Dataset },
type = { Open Source Dataset },
author = { AI MODEL V2 },
howpublished = { \url{ https://universe.roboflow.com/ai-model-v2/object-detection-model-for-identification-of-poisonous-plants-in-the-chesapeake-bay-watershed-p8o4b } },
url = { https://universe.roboflow.com/ai-model-v2/object-detection-model-for-identification-of-poisonous-plants-in-the-chesapeake-bay-watershed-p8o4b },
journal = { Roboflow Universe },
publisher = { Roboflow },
year = { 2023 },
month = { jun },
note = { visited on 2025-02-16 },
}