Hyper-Kvasir Computer Vision Project
Updated 4 months ago
2.9k
views80
downloadsMetrics
Overview This is the largest Gastrointestinal dataset generously provided by Simula Research Laboratory in Norway
You can read their research paper here in Nature
In total, the dataset contains 10,662 labeled images stored using the JPEG format. The images can be found in the images folder. The classes, which each of the images belong to, correspond to the folder they are stored in (e.g., the ’polyp’ folder contains all polyp images, the ’barretts’ folder contains all images of Barrett’s esophagus, etc.). Each class-folder is located in a subfolder describing the type of finding, which again is located in a folder describing wheter it is a lower GI or upper GI finding. The number of images per class are not balanced, which is a general challenge in the medical field due to the fact that some findings occur more often than others. This adds an additional challenge for researchers, since methods applied to the data should also be able to learn from a small amount of training data. The labeled images represent 23 different classes of findings.
The data is collected during real gastro- and colonoscopy examinations at a Hospital in Norway and partly labeled by experienced gastrointestinal endoscopists.
Use Cases
"Artificial intelligence is currently a hot topic in medicine. The fact that medical data is often sparse and hard to obtain due to legal restrictions and lack of medical personnel to perform the cumbersome and tedious labeling of the data, leads to technical limitations. In this respect, we share the Hyper-Kvasir dataset, which is the largest image and video dataset from the gastrointestinal tract available today."
"We have used the labeled data to research the classification and segmentation of GI findings using both computer vision and ML approaches to potentially be used in live and post-analysis of patient examinations. Areas of potential utilization are analysis, classification, segmentation, and retrieval of images and videos with particular findings or particular properties from the computer science area. The labeled data can also be used for teaching and training in medical education. Having expert gastroenterologists providing the ground truths over various findings, HyperKvasir provides a unique and diverse learning set for future clinicians. Moreover, the unlabeled data is well suited for semi-supervised and unsupervised methods, and, if even more ground truth data is needed, the users of the data can use their own local medical experts to provide the needed labels. Finally, the videos can in addition be used to simulate live endoscopies feeding the video into the system like it is captured directly from the endoscopes enable developers to do image classification."
Borgli, H., Thambawita, V., Smedsrud, P.H. et al. HyperKvasir, a comprehensive multi-class image and video dataset for gastrointestinal endoscopy. Sci Data 7, 283 (2020). https://doi.org/10.1038/s41597-020-00622-y
Using this Dataset
Hyper-Kvasir is licensed under a Creative Commons Attribution 4.0 International (CC BY 4.0) License, which permits use, sharing, adaptation, distribution and reproduction in any medium or format, as long as you give appropriate credit to the original author(s) and the source. This means that in all documents and papers that use or refer to the Hyper-Kvasir dataset or report experimental results based on the dataset, a reference to the related article needs to be added: PREPRINT: https://osf.io/mkzcq/. Additionally, one should provide a link to the Creative Commons license, and indicate if changes were made. The images or other third party material in this article are included in the article’s Creative Commons license, unless indicated otherwise in a credit line to the material. If material is not included in the article’s Creative Commons license and your intended use is not permitted by statutory regulation or exceeds the permitted use, you will need to obtain permission directly from the copyright holder. To view a copy of this license, visit http://creativecommons.org/licenses/by/4.0/
About Roboflow
Roboflow makes managing, preprocessing, augmenting, and versioning datasets for computer vision seamless.
Developers reduce 50% of their boilerplate code when using Roboflow's workflow, automate annotation quality assurance, save training time, and increase model reproducibility.
Use This Trained Model
Try it in your browser, or deploy via our Hosted Inference API and other deployment methods.
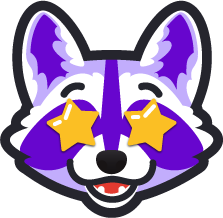
Build Computer Vision Applications Faster with Supervision
Visualize and process your model results with our reusable computer vision tools.
Cite This Project
If you use this dataset in a research paper, please cite it using the following BibTeX:
@misc{
hyper-kvasir_dataset,
title = { Hyper-Kvasir Dataset },
type = { Open Source Dataset },
author = { Simula },
howpublished = { \url{ https://universe.roboflow.com/simula/hyper-kvasir } },
url = { https://universe.roboflow.com/simula/hyper-kvasir },
journal = { Roboflow Universe },
publisher = { Roboflow },
year = { 2024 },
month = { jul },
note = { visited on 2024-11-21 },
}