RSDD Computer Vision Project
Updated 17 hours ago
2.7k
views118
downloadsDescription
Potholes are a common problem in damaged roads, where people stumble, vehicles get damaged, and drivers lose control over their cars. The maintenance of roads is a costly necessity that developing countries' authorities often struggle to deliver in time. The present dataset was collected to develop a prioritisation system that combines deep learning models and traditional computer vision techniques to automate the analysis of road irregularities reported by citizens [1]. Although the images in the dataset come from different sources (e.g. web scraping), we attribute the authorship of a main portion to the well-known RDD2020 dataset [2]. For the labelled images, we enhanced the original annotations by relabelling and focusing on four categories: crocodile cracks, lateral cracks, longitudinal cracks and potholes. We iteratively filtered bad samples and improved the annotations. As a result, the resulting object detection models have allowed us to better discriminate road damage severity, in contrast to just detecting potholes. Here are a few use cases for this dataset:
-
Automated Road Inspection: Researchers working on transportation and mobility issues could use the RSDD dataset to train models and embed them inside UAVs and road quality survey vehicles to make road damage inspection more efficient. Most cities in the world inspect failures in person and rely on manual record-keeping. Developing an edge-AI-based system could help automatically detect and log road damage on longer roads, improving road damage maintenance prioritisation.
-
Road Maintenance and Repair: Municipalities and public works departments could use the RSDD dataset to train models to analyse road conditions server-side, prioritising repair activities by identifying more serious damage such as crocodile cracks and potholes.
-
Autonomous Vehicles: Developers in the autonomous vehicle industry could utilize the RSDD dataset to enhance the situational awareness capability of their vehicles. By recognising road damage, AI systems could make more informed navigation decisions, enhancing safety and efficiency.
[1] E. Salcedo, M. Jaber, and J. Requena Carrión, “A novel road maintenance prioritisation system based on computer vision and crowdsourced reporting,” Journal of Sensor and Actuator Networks, vol. 11, no. 1, p. 15, 2022. doi:10.3390/jsan11010015
[2] D. Arya, H. Maeda, S. K. Ghosh, D. Toshniwal, and Y. Sekimoto, “RDD2020: An annotated image dataset for Automatic Road Damage Detection Using Deep Learning,” Data in Brief, vol. 36, p. 107133, 2021. doi:10.1016/j.dib.2021.107133
Citation
If you use this dataset in your research, please considering citing the following work:
@article{Salcedo_Jaber_Requena Carrión_2022, title={A novel road maintenance prioritisation system based on computer vision and crowdsourced reporting}, volume={11}, DOI={10.3390/jsan11010015}, number={1}, journal={Journal of Sensor and Actuator Networks}, author={Salcedo, Edwin and Jaber, Mona and Requena Carrión, Jesús}, year={2022}, pages={15}}
You are also encourage to explore the original dataset provided by D. Arya et al.
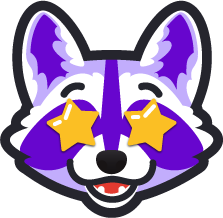
Build Computer Vision Applications Faster with Supervision
Visualize and process your model results with our reusable computer vision tools.
Cite This Project
If you use this dataset in a research paper, please cite it using the following BibTeX:
@misc{
rsdd_dataset,
title = { RSDD Dataset },
type = { Open Source Dataset },
author = { RDMO },
howpublished = { \url{ https://universe.roboflow.com/rdmo/rsdd } },
url = { https://universe.roboflow.com/rdmo/rsdd },
journal = { Roboflow Universe },
publisher = { Roboflow },
year = { 2024 },
month = { dec },
note = { visited on 2024-12-26 },
}